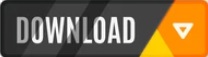
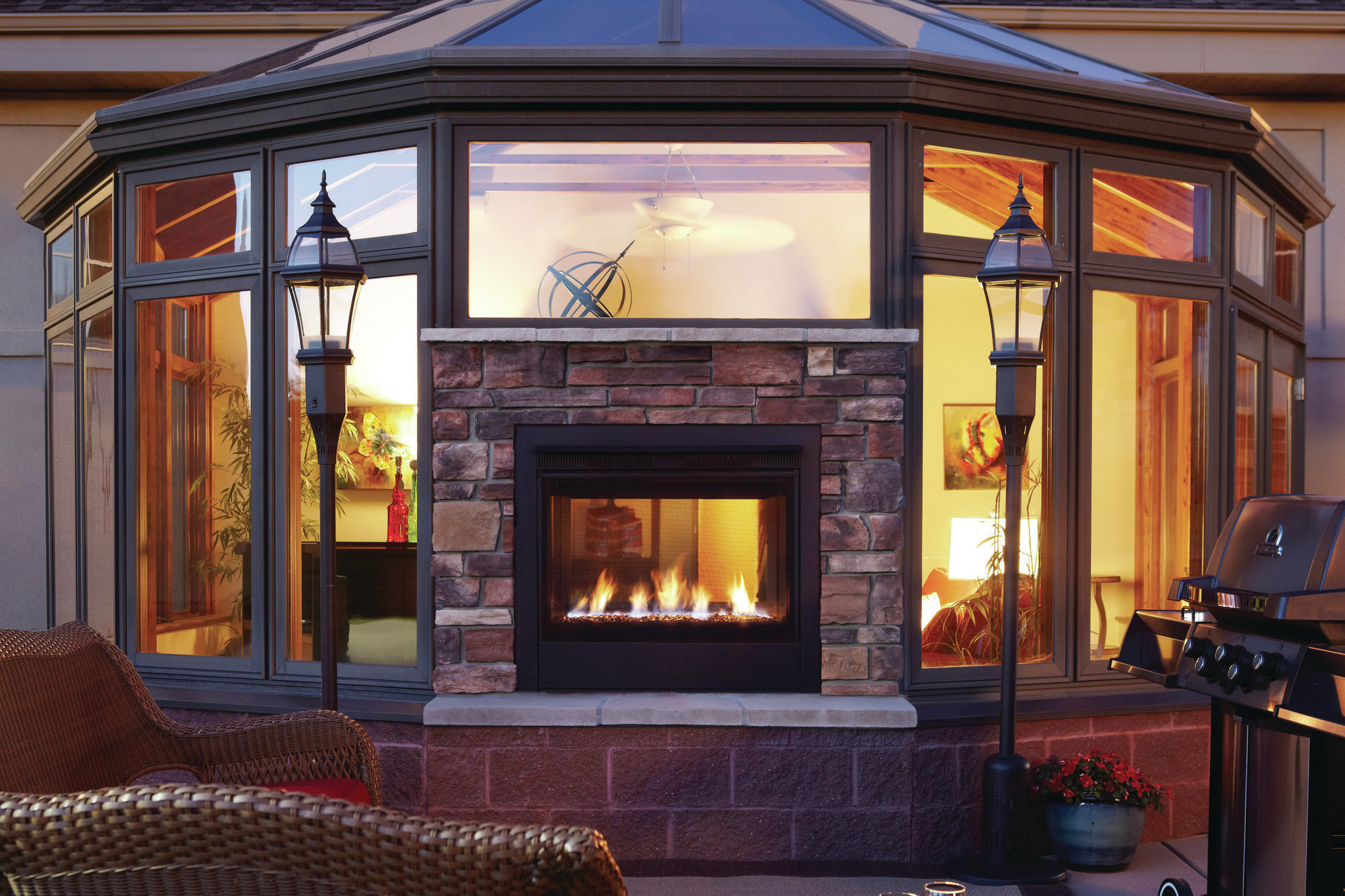
Here well use two concrete examples to illustrate partial derivatives: first well look at a curve y(x) that is also a function of time, i.e.
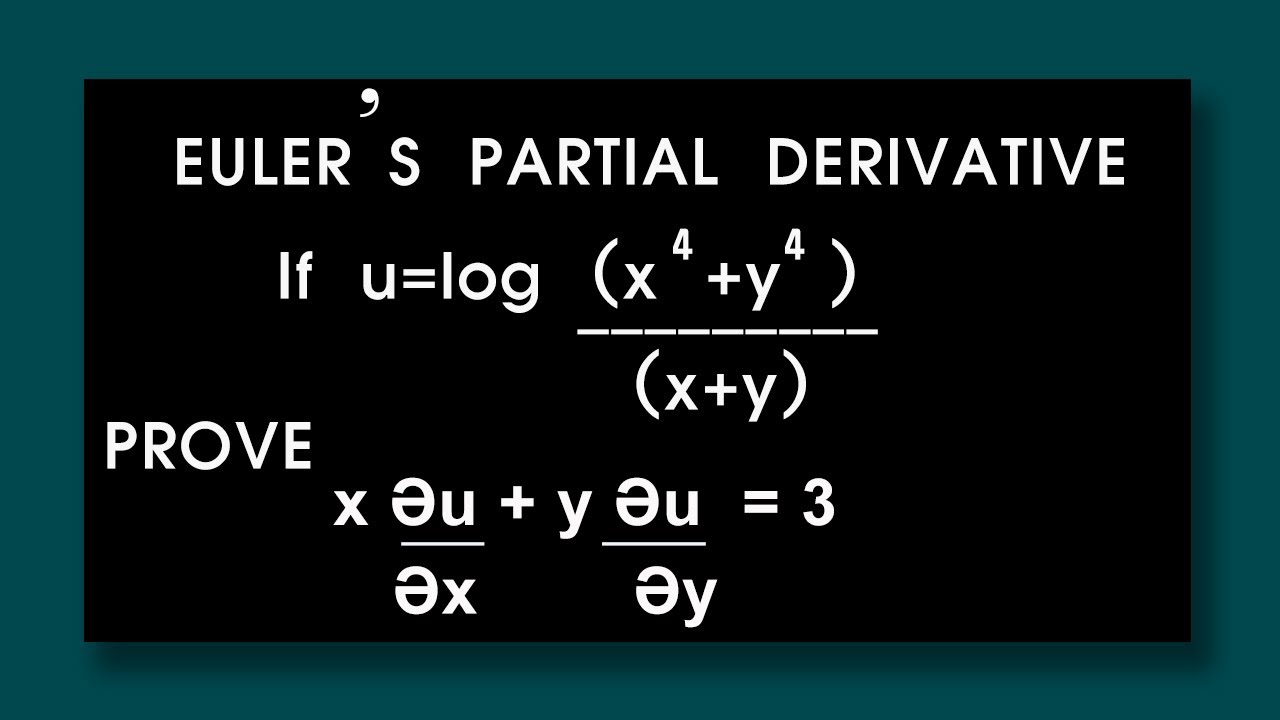
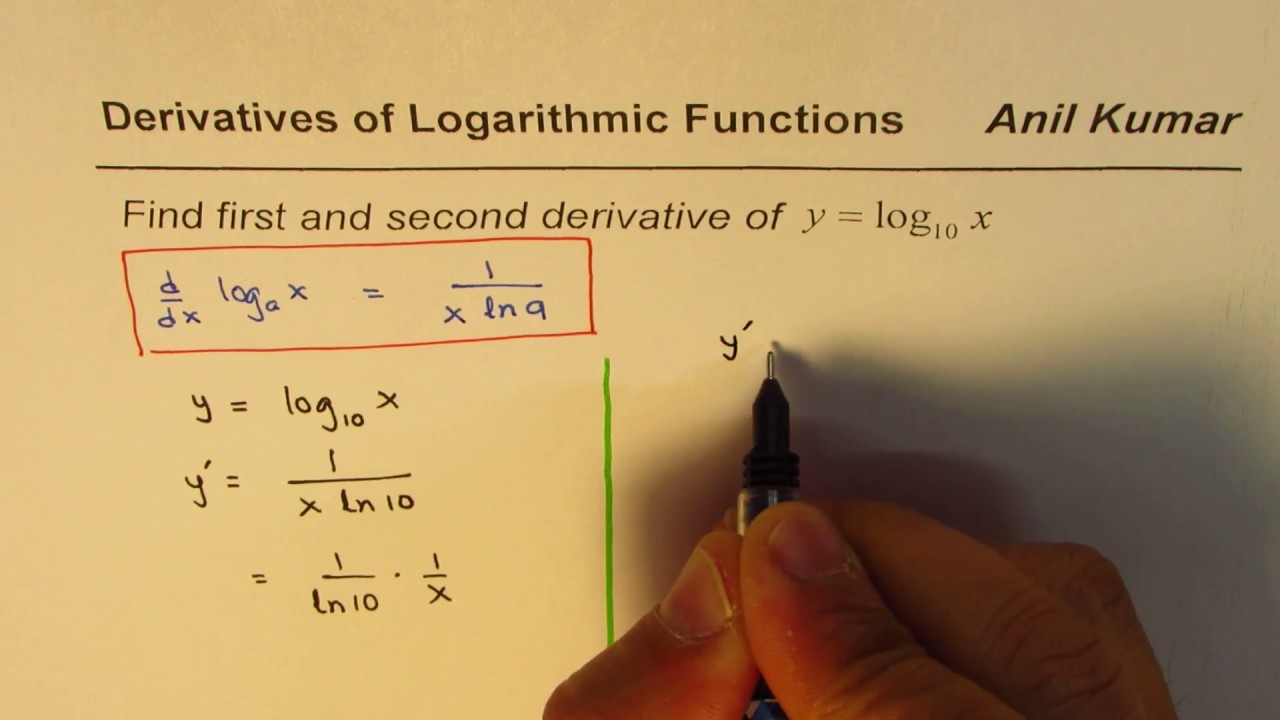
( z j)) In classification problem, the n here represents the number of classes, and y j is the one-hot representation of the actual class. This can be written as: CE j 1 n ( y j log. LIkewise, when you differentiate with respect to #y#, you treat #x# and #z# as constants, and so on. In two dimensions, when we have a function y(x), we can readily define dy/dx as the slope of the curve y(x). Another common task in machine learning is to compute the derivative of cross entropy with softmax. As you will see if you can do derivatives of functions of one variable you won’t have much of an issue with partial derivatives.
Partial derivative of log how to#
We will give the formal definition of the partial derivative as well as the standard notations and how to compute them in practice (i.e. When you differentiate with respect to #x#, you treat #y# and #z# as constants. In this section we will the idea of partial derivatives. Now to find action as a function of time, we have to fix final position x ( t 2) x 2 and rewrite x in terms of it. Since you're dealing with a multivariable function, you must treat #x#, #y#, and #z# as independent variables and calculate the partial derivative of #w#, your dependent variable, with respect to #x#, #y#, and #z#. Solving the corresponding equations of motion with initial position x ( 0) 0, well find that true motion is described by x a sin.
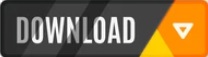